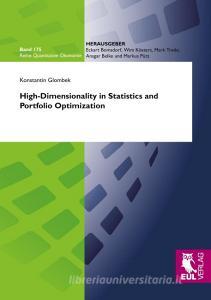
High-Dimensionality in Statistics and Portfolio Optimization
- Editore:
Josef Eul Verlag GmbH
- Collana:
- Quantitative Ökonomie, Bd. 175
- EAN:
9783844102130
- ISBN:
3844102132
- Pagine:
- 148
- Formato:
- Paperback
- Lingua:
- Tedesco
Descrizione High-Dimensionality in Statistics and Portfolio Optimization
Many challenges in multivariate analysis face the problem of dealing with samples whose dimension is of the same order as their size. This high-dimensional setting often leads to inconsistencies or degenerated distributions of certain estimators. In particular, estimators which are based on the sample covariance matrix are affected as the eigenvalues of this matrix behave differently under high-dimensionality than the ones of the population covariance matrix. But the eigenvalues of certain estimators for scatter also exhibit a remarkable behavior in the classical setting when the sample size is much larger than the dimension. The first major contribution of this thesis is the establishment of the semicircle law of Tyler's M-estimator for scatter. It is shown that the empirical distribution of the eigenvalues of this estimator, suitably standardized, converges in probability to the semicircle law under spherical sampling and assuming that the sample dimension and size tend to infinity while their ratio tends to zero. The second focus of this thesis is on covariance matrix testing. A completely new test for a scalar multiple of the covariance matrix of a normal population under high-dimensionality is derived. This new test is motivated by the properties of the semicircle law in free probability theory and exhibits large local power if the ratio of dimension to sample size is small. Statistical inference for high-dimensional portfolios is the third contribution of this thesis. The standard estimators for the variance and mean of the portfolio return of the global minimum variance, naive and tangency portfolio are investigated concerning consistency and asymptotic distribution under high-dimensionality. The corresponding Sharpe ratios and the weights of the global minimum variance portfolio are considered as well. An application to financial data illustrates the results.