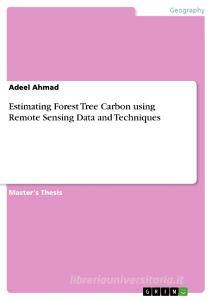
Estimating Forest Tree Carbon using Remote Sensing Data and Techniques
- Editore:
GRIN Verlag
- EAN:
9783668500266
- ISBN:
3668500266
- Pagine:
- 84
- Formato:
- Paperback
- Lingua:
- Tedesco
Descrizione Estimating Forest Tree Carbon using Remote Sensing Data and Techniques
Master's Thesis from the year 2013 in the subject Geography / Earth Science - Physical Geography, Geomorphology, Environmental Studies, grade: 3.87, University of the Punjab (Institute of Geology), course: Geomatics, language: English, abstract: Alarming rates of carbon increase in the atmosphere threatens our planet for sustainability. For avoiding carbon release and to sequester the carbon emissions from the forests and other sources, nations of the world are striving hard to design methodologies for monitoring carbon emission and sequester rates in the forests and other carbon pools. This book presents a research based on a very modern approach to estimate carbon in the moist temperate Himalayan forest of Ayubia National Park (ANP), Pakistan. Latest Geospatial techniques including Remote Sensing and Geographical Information System (GIS) were incorporated to estimate carbon in the forest of ANP using the up-to-date statistical approaches. Objectives of this research included landcover mapping of the study area and estimating and mapping the carbon in the forest as well as biomass for the same area. High spatial resolution Satellite Pour I¿Observation de la Terre-5 (SPOT-5) satellite imagery is used to map the landcover of the study area. Different bands from Landsat-5 Thematic Mapper (TM) and SPOT-5 satellite images have been incorporated with inclusion of topographic factors to estimate the carbon stock. The results showed that three landcover types of ANP forest including mix forest, conifer forest and shadow conifer forest have 104,781.62, 445,290.61 and 301,972.37 tons of total aboveground carbon (AGC) respectively as calculated from field inventory data with an average of 252.26 tC/Ha as compared to the previously calculated average of AGC of 222.99 tC/Ha at ANP. This research concluded that multiple and non-linear regression models can estimate carbon more accurately as compared to linear regression models. Linear regression correlations were poor while the Multiple Regression Analysis (MRA) method showed coefficient of correlation (R2) value 0.901 Random Forests (RF) prediction modeling showed R2 value 0.90 while Classification And Regression Tree (CART) explained R2 value 0.72. This proved that multiple and non-linear regression data mining models, such as MRA and RF, are best suitable while working with remote sensing techniques in natural systems like forests to calculate AGC.